Deep learning tool helps NASA discover 301 exoplanets
NASA scientists used a neural network called ExoMiner to examine data from Kepler, increasing the total tally of confirmed exoplanets in the universe.
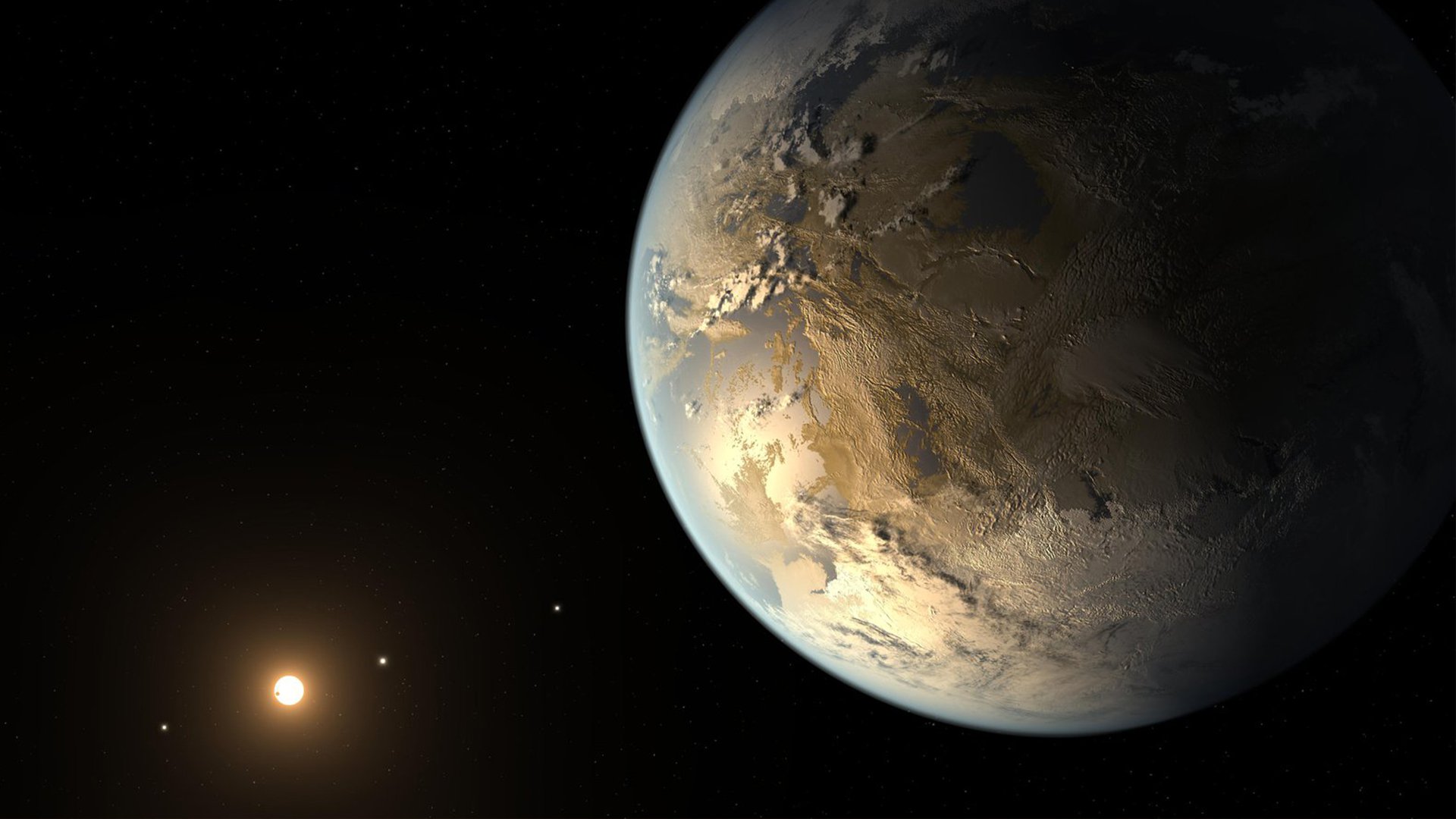
An artist's concept of exoplanet Kepler-186f. Discovered by Kepler in 2014, Kepler-186f is the first validated Earth-size planet to orbit a distant star in the habitable zone. Image Credit: NASA/JPL
Scientists just added 301 exoplanets to an already confirmed cohort of more than 4,000 worlds outside our solar system.
Most exoplanets known to scientists have been discovered by NASA’s Kepler spacecraft, which was retired in October 2018 after nine years of collecting data from deep space. Kepler, which as of its retirement had discovered more than 2,600 exoplanets, “revealed our night sky to be filled with billions of hidden planets—more planets even than stars,” NASA reports in a press release. Kepler would look for temporary dimness in the stars it was observing, a sign that a planet may be moving in front of it from the spacecraft’s perspective. The easiest planets to detect have been gas giants like Saturn and Jupiter. But scientists have also been able to use data from Kepler to identify Earth-like planets in the habitable zone, an area around a star that’s neither too hot nor too cold for liquid water to exist on a planet.
The challenge scientists have historically faced is a time-related one: “For missions like Kepler, with thousands of stars in its field of view, each holding the possibility to host multiple potential exoplanets, it's a hugely time-consuming task to pore over massive datasets,” NASA reported on November 22 in a press release. So, when it came to identifying the latest 301 exoplanets, researchers based at NASA’s Ames Research Center in Mountain View, California, turned to a new deep neural network called ExoMiner.
Now, in a paper accepted for publication in The Astrophysical Journal, the team describes how, analyzing data from NASA’s Pleiades supercomputer, ExoMiner was able to identify planets outside our solar system. It did so by parsing through data from Kepler and the spacecraft’s second mission K2, distinguishing “real exoplanets from different types of imposters, or ‘false positives,’” NASA reports.
The Kepler Science Operations Center pipeline originally identified the 301 exoplanets, which were then promoted to planet candidates by the Kepler Science Office before being officially confirmed as exoplanets by ExoMiner, NASA reports.
ExoMiner “is a so-called neural network, a type of artificial intelligence algorithm that can learn and improve its abilities when fed a sufficient amount of data,” Tereza Pultarova writes for Space.com. Its technology is based on exoplanet-identification techniques used by scientists. To test its accuracy, the team gave ExoMiner a test set of exoplanets and potential false positives, and it successfully retrieved 93.6% of all exoplanets. The neural network “is considered more reliable than existing machine classifiers” and, given human biases and error, “human experts combined,” Marcia Sekhose writes for Business Insider India.
"When ExoMiner says something is a planet, you can be sure it's a planet," ExoMiner Project Lead Hamed Valizadegan told NASA.
But the neural network does have some limitations. It “sometimes fails to adequately utilize diagnostic tests,” including a centroid test, which identifies large changes in a center of a star as an object passes by it, the researchers report in the paper. And at the time of the study, ExoMiner did not have the data required to decode “flux contamination,” a measurement of contaminants coming from a source. (In the hunt for exoplanets, flux contamination often refers to the light of a star in the background or foreground of a target star interfering with data coming from the target star.) Finally, ExoMiner and other data-driven models using visible light to detect exoplanets cannot correctly classify giant exoplanets orbiting orange dwarf stars. But these giant planet candidates are incredibly rare in Kepler data, the researchers report.
Because they exist outside the habitable zones of their stars, Pultarova writes, none of the 301 exoplanets identified by ExoMiner are likely to host life. But soon, scientists will use ExoMiner to tackle data from other exoplanet hunters, including NASA’s Transiting Exoplanet Survey Satellite (TESS). Unlike Kepler, which surveyed star systems 600 to 3,000 light-years away before running out of fuel, TESS, which launched six months before Kepler’s end, documents stars and their exoplanets within 200 light-years from Earth. These nearby exoplanets are the ripest for scientific exploration, scientists believe.
“With a little fine-tuning,” the NASA Ames team can transfer ExoMiner’s learnings from Kepler and K2 to other missions like TESS, Valizadegan told NASA. “There’s room to grow,” he said.