With ‘Problems Out There Waiting for Us,’ Public Health Practitioners Turn to AI for Help
Scarce funding, ethical concerns, and other problems have limited the use of artificial intelligence to tackle public health issues. That’s starting to change.
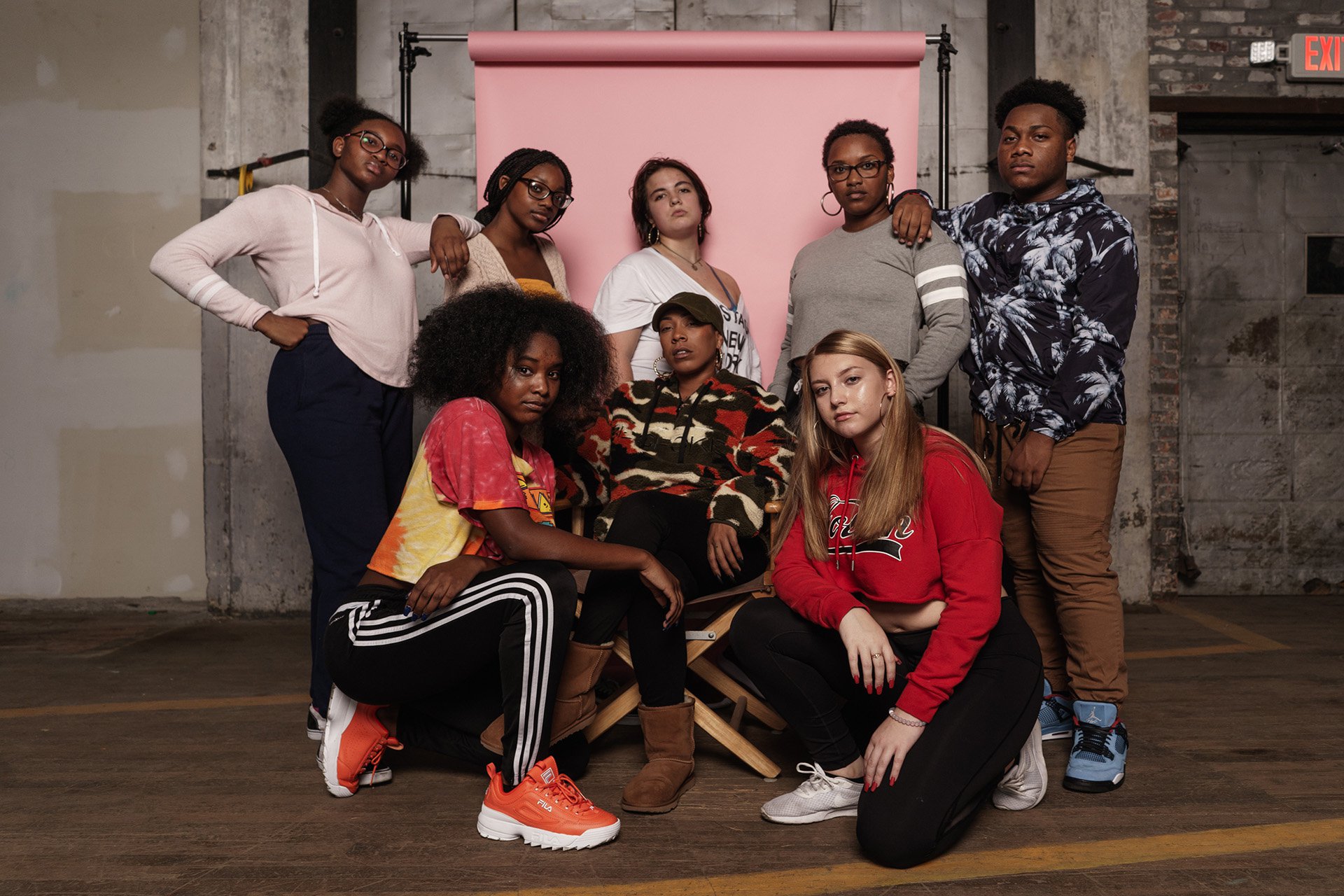
These dancers from a Syracuse-based dance studio helped with the design and branding of the AI-powered chatbot Layla. Image credit: Tiffany Lloyd, Layla
When Yanira was a teenager, she didn’t feel like she could ask her parents about sex. So like most kids her age, she would try looking up answers to her questions online—only to get stuck looking up the medical terms some sites used, or trying to sort out whether the source was legitimate or not.
Now Yanira is 20 years old, and her 15-year-old sister is turning to her with questions. But Yanira doesn’t have to Google anything anymore: She sends her sister to a chatbot named Layla, an AI-powered tool built by a public health nonprofit for the girls of Syracuse, New York.
“It was a lot better than I could have thought of off the top of my head," she says of the information provided by Layla.
Yanira knew about the site because she had been part of a group of young women to give feedback on the chatbot. She liked the way it responded to questions, and thought it made answers clear. She also liked that it provided links to where to get real-world services in her area.
“When I was 15, I didn’t know much about birth control, much less where to get it," Yanira says.
Teen pregnancy is a real problem in Syracuse. For girls between ages 15 and 17, the rate is 21.8 per 1,000, compared to 18.8 in the U.S. as a whole. And for black and Hispanic teenagers, the rates are much higher: 65.6 and 53.3 per 1,000, respectively. A study found that 80 percent of those pregnancies were unplanned.
That same research, conducted by Public Good Projects (PGP), the public health nonprofit behind the chatbot, found that a major cause behind the high pregnancy rates was these young women’s limited understanding of sex.
“What does a condom look like? They couldn’t tell you," says Joe Smyser, the CEO of PGP.
Finding useful, accurate information can be hard for teens. Random internet searches run the risk of finding incorrect information, while reputable sources can be written in dry language that doesn’t reflect the lived experience of teenage girls. Going to parents isn’t always an option, either.
But Layla is available 24/7, and no question is off-limits. As the opening prompt says, Layla wants to be considered “your new best friend with all the deets about those uncomfortable topics like birth control, sex, and STDs.”
PGP enlisted a group of young women from Syracuse to help them design the chatbot, which uses IBM’s Watson technology—specifically its natural language processing abilities—to make the chatbot nimbler at answering questions phrased in a variety of ways. Although cutting edge AI powers the site, everything from the resources it recommends, to the language it uses, to its aesthetic look and feel were designed by local teenagers who need it most.
“We didn’t want this to come off like a healthcare department,” says Tiffany Lloyd, Layla’s community manager. “It was created by brown girls, for brown girls.”
Jason Wagner, 16, and Deziah Thomas, 15, are two youth influencers that helped to promote Layla, a chatbot designed to help young people of color get reliable information about sexual health, including where to find additional resources and services. Image credit: Tiffany Lloyd, Layla
AI for the Globe
The field of public health has the ambitious goal of preventing the spread of diseases and extending life across human populations that span everything from small communities to entire continents. It’s a daunting challenge with new problems constantly cropping up, often with insufficient resources to tackle them. Those challenges have led public health workers to look to AI to find novel ways to solve global health issues like tuberculosis and teenage pregnancy.
One of the earliest and best-known attempts to use big data for public health—done before AI research really took off—was predicting the spread of flu from Google searches. While Google has ended its internally run research, the company has added new AI capabilities and still passes data along so that places like Boston Children's Hospital can continue the work. By going through electronic health records, flu-related Google searches, and historical flu activity in a given location, the AI-powered tool has demonstrated the ability to spot flu outbreaks two weeks before the Centers for Disease Control (CDC) can.
This approach is not entirely new. Disease surveillance systems now routinely track people researching symptoms in Google searches, as well as more traditional, expensive-to-gather clinical data. But more sophisticated data crunching algorithms exist for marketers: Layla developer PGP, for example, used a tool originally designed to monitor “brand health,” a mix of various metrics companies look at to see how their company is perceived by the public, and repurposed it for tracking opioid use and mental health trends.
“No one in academia understands Twitter the way marketers do,” Smyser says.
With data from a wide range of traditional and social media sources, including closed captioning from local television, newspapers, Facebook, Twitter, Instagram, and YouTube, PGP is generating new insights into opioid use. But it’s only possible with natural language processing, a subset of AI that parses written or spoken word—like the Layla chatbot does with IBM’s Watson. In this instance, the AI learns to find stories and tweets about opioids, even if slang is used, without having to be taught every different way the drug use might be referenced.
Right now, the system picks up about 55,000 references to opioids a day. These might include everything from a tweet from President Trump boasting of a fentanyl bust to Facebook posts of dealers hawking a product. With such a large stream of data, experts are able to conduct a lot of previously out-of-reach analysis. Smyser is sharing what PGP is learning with presentations to agents from the Drug Enforcement Agency (DEA) and community groups, but there are limits to how much small, community-based nonprofits like his can help.
“There is no red phone for me to pick up and tell CDC they need to be aware of this,” Smyser says.
Old Methods, New AI Insights
Other public health AI projects don’t rely on internet data. There are nearly 2 million homeless young people between 13 and 24 in the United States, so researchers out of the University of Southern California’s Center for AI in Society (CAIS) developed software called TAY Triage Tool to combat the problem. It uses information from a short questionnaire to predict which young people are at risk of long-term homelessness, allowing decision-makers to divert precious resources to those most in need.
A similar tool called HEALER figures out who in a group of homeless youth is the best person to train about HIV prevention. The traditional approach is to bring in and train the most popular person. But HEALER actually discovers different members of the group with more widespread connections than the most popular individuals, who typically hang out with smaller groups.
“We are trying to train gatekeepers to watch over others in their social networks,” says Milind Tambe, founding co-director of CAIS and an engineering professor at USC, who worked on both projects.
Sometimes, AI algorithms just confirm best practices and the cost-effectiveness of solutions, always a priority for cash-strapped public health programs. There are over two million individuals with tuberculosis in India, and treating it presents a major challenge: Multi-drug resistant (MDR) tuberculosis is widespread and hard to diagnose at scale.
“Finding these strains of tuberculosis is really important,” says Sze-chuan Suen, a professor of engineering at USC.
GeneXpert is a test that can detect drug-resistant bacteria—but it does at a high cost. Suen did a study on this expensive screening and concluded, despite GeneXpert being costlier in the short term than other methods, it should be used at initial diagnosis for all patients. The benefits of identifying patients with drug-resistant strains of TB should save money—and lives—in the long run.
Part of a Balanced Global Diet
Compared to industries like healthcare or education, public health has not had a massive influx of AI projects. In part, that’s because much of the progress in AI has been around image recognition, which can be most readily applied to individual medical diagnoses. For example, Stanford researchers reported in 2017 that an artificial neural network they built could diagnose dangerous melanomas from photographs as accurately as experienced dermatologists, which could expand access to care in areas with fewer specialists.
Another factor limiting AI applications is the small budgets and profits to be made in public health, which lowers the likely return on investment in new technologies. And many public health projects go through ethics boards that determine the rules around use of public data and research protocols. If anything, these processes “can be cautious to a fault” and delay approval, according to Henry Kautz, a computer science professor at the University of Rochester.
That doesn’t mean AI won’t be utilized in public health in the near future in a significant way. The problems are simply too big, and AI’s potential too enticing, not to try.
“There are problems out there waiting for us,” Tambe says.
Another challenge in applying AI to public health is the field’s focus on whole populations, not individuals. Unlike other industries that create tools that individuals can put into use—such as apps for recognizing farm pests or personalized learning tools—public health demands solutions for broad populations with an inherently wider set of needs. This often means that data and algorithms can only take us so far before humans must get involved.
Take foodborne illnesses: AI algorithms can sift quickly through large datasets to spot problems, but that’s only half the battle—government inspectors must then ground truth the findings with in-person inspections.
For the chatbot Layla, that means providing phone numbers to real people for further help for search queries that require follow up, like where to get STD testing or services for sexual assault victims. Lloyd, Layla’s community manager, says that more outreach work needs to be done to understand and address issues that are too nuanced for current AI.
“Make no mistake, the chatbot is not the end-all,” Lloyd says.
Lloyd continues to connect with the young women Layla is meant to serve in focus groups, and makes sure the word gets out with social media campaigns and posters at places like hair salons.
“They need an ongoing conversation,” Lloyd says.
Still, for underserved populations like teenage girls in Syracuse, AI like Layla has its place and has room to grow. Yanira had suggested other capabilities for the site, like a period tracker. In the meantime, Yanira says she would recommend it to friends and even her two other little sisters.
“It is kind of like another big sister,” she says.